Data assimilation systems can provide accurate initial fields for further improving numerical weather prediction (NWP). Since 2008, TIAN Xiangjun and his team at the Institute of Atmospheric Physics, Chinese Academy of Sciences, have devoted considerable effort to developing the nonlinear least-squares 4-D ensemble variational data assimilation method (NLS-4DVar). NLS-4DVar is now quite advantageous and has great potential because of its ease of implementation and the absence of any need to invoke the tangent and adjoint models, as well as offering superior performance. Due to its prominent advantages, the NLS-4DVar methods have been used for solving real-world applications including land data assimilation, NWP data assimilation, atmospheric-chemistry data assimilation, and targeted observations.
TIAN’s team developed a new forecasting system—the System of Multigrid Nonlinear Least-squares Four-dimensional Variational (NLS-4DVar) Data Assimilation for Numerical Weather Prediction (SNAP). SNAP is built upon the multigrid NLS-4DVar data assimilation scheme, the operational Gridpoint Statistical Interpolation (GSI)–based data-processing and observation operators, and the widely used Weather Research and Forecasting numerical model.
The multigrid NLS-4DVar assimilation framework is used for the analysis, which can adequately correct errors from large to small scales and accelerate iteration solutions. The analysis variables are model state variables, rather than the control variables adopted in the conventional 4DVar system. Currently, the team has achieved the assimilation of conventional and radar observations, and will continue to improve the assimilation of satellite observations in the near future.
"We carefully designed several groups of real experiments, including one case and one-week cycling assimilation experiments, in order to comprehensively evaluate SNAP in this study," TIAN introduced their study recently published in Advances in Atmospheric Sciences. The numerical results demonstrated that, in terms of the precipitation intensity, TS [threat score] and ETS [equitable threat score], SNAP can fully absorb observations and improve the initial fields, thereby improving the precipitation forecast. In particular, compared with GSI 4DEnVar, SNAP produces slightly lower forecast RMSEs [root-mean-square errors] and more positive RPI [relative percentage improvement] as a whole.
"The emergence of SNAP provides a promising way with a sound theoretical basis for data assimilation in NWP to significantly improve the forecast skills in an era where the number of observations, especially from remote sensing techniques, is significantly increasing," explains TIAN. "It is of great importance and practical application to explore more sophisticated data assimilation methods and systems for improving the precision of both weather prediction and climate predictions in the big data era."
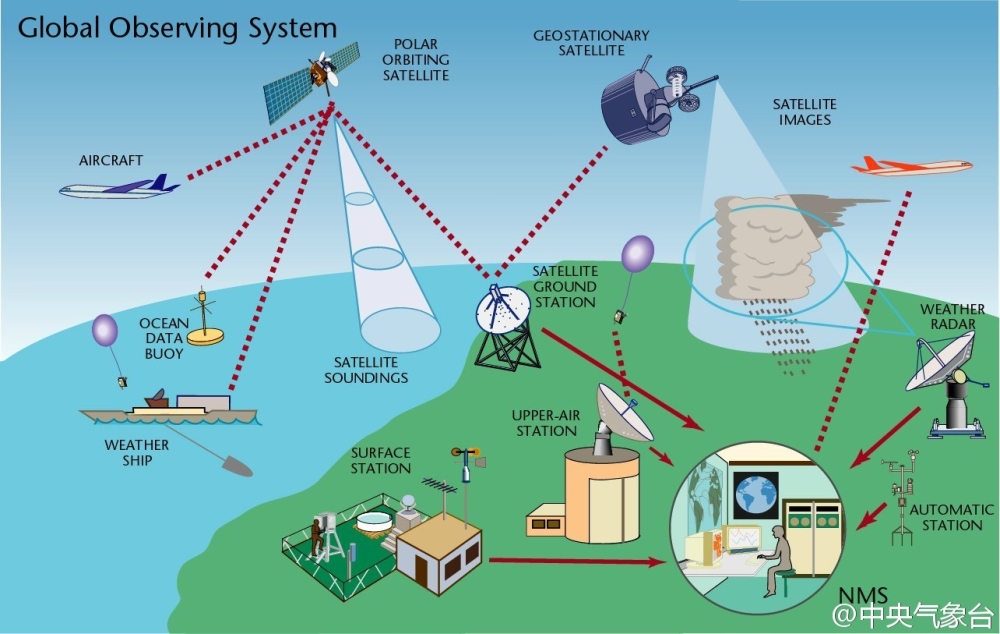
The Global Observing System (http://blog.sina.com.cn/s/blog_781c1bd70102vv1l.html)
This work was partially supported by the National Key Research and Development Program of China (Grant No. 2016YFA0600203), the National Natural Science Foundation of China (Grant No. 41575100), the Key Research Program of Frontier Sciences, Chinese Academy of Sciences (Grant No.QYZDY-SSW-DQC012) and the CMA Special Public Welfare Research Fund (Grant No. GYHY201506002).
Citation: Zhang, H. Q., X. J. Tian, W. Cheng, and L. P. Jiang, 2020: System of Multigrid Nonlinear Least-squares Four dimensional Variational Data Assimilation for Numerical Weather Prediction (SNAP): System formulation and preliminary evaluation. Adv. Atmos. Sci., 37(11), https://doi.org/10.1007/s00376-020-9252-1.
Media contact: Ms. LIN Zheng, jennylin@mail.iap.ac.cn