Many climate-related studies, such as detection and attribution of historical climate change, projections of future climate and environments, and adaptation to future climate change, heavily rely on the performance of climate models. Concisely summarizing and evaluating model performance becomes increasingly important for climate model intercomparison and application, especially when more and more climate models participate in international model intercomparison projects.
Most of current model evaluation metrics, e.g., root mean square error (RMSE), correlation coefficient, standard deviation, measure the model performance in simulating individual variable. However, one often needs to evaluate a model’s overall performance in simulating multiple variables. To fill this gap, an article published in Geosci. Model Dev., presents a new multivariable integrated evaluation (MVIE) method.
"The MVIE includes three levels of statistical metrics, which can provide a comprehensive and quantitative evaluation on model performance.” Says XU, the first author of the study from the Institute of Atmospheric Physics, Chinese Academy of Sciences. The first level of metrics, including the commonly used correlation coefficient, RMS value, and RMSE, measures model performance in terms of individual variables. The second level of metrics, including four newly developed statistical quantities, provides an integrated evaluation of model performance in terms of simulating multiple fields. The third level of metrics, multivariable integrated evaluation index (MIEI), further summarizes the three statistical quantities of second level of metrics into a single index and can be used to rank the performances of various climate models. Different from the commonly used RMSE-based metrics, the MIEI satisfies the criterion that a model performance index should vary monotonically as the model performance improves.
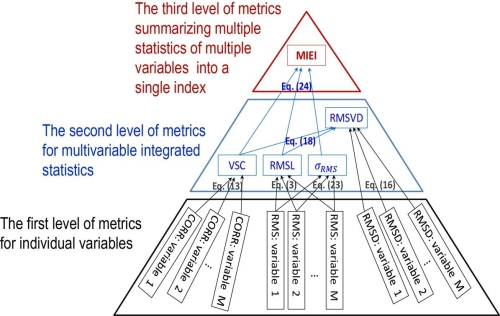
Pyramid chart showing the relationship between three levels of metrics for multivariable integrated evaluation of climate model performance. (Image by XU Zhongfeng)
According to the study, higher level of metrics is derived from and concisely summarizes the lower level of metrics. “Inevitably, the higher level of metrics loses detailed statistical information in contrast to the lower level of metrics.” XU therefore suggests, "To provide a more comprehensive and detailed evaluation of model performance, one can use all three levels of metrics.”
Reference:
Xu Zhongfeng, Han Ying, and Fu Congbin, 2017: Multivariable integrated evaluation of model performance with the vector field evaluation diagram, Geosci. Model Dev., 10, 3805–3820. https://www.geosci-model-dev.net/10/3805/2017
Xu Zhongfeng, Hou Zhaolu, Han Ying, and Guo Weidong, 2016: A diagram for evaluating multiple aspects of model performance in simulating vector fields, Geosci. Model Dev., 9, 4365–4380. www.geosci-model-dev.net/9/4365/2016/
Media contact: Ms. LIN Zheng, jennylin@mail.iap.ac.cn